“Any sufficiently advanced technology is indistinguishable from magic”.
Arthur C. Clarke’s famous aphorism has enjoyed for many years absolute validity among most of society regarding biometric identity verification technologies. We have all fascinated by scenes in series and films in which criminals were quickly recognized by an image taken from a security camera, a phone call or a fingerprint. Biometric profiles were compared in large touch screens at full speed until the expected match arrived, and in the end always the same question: is it possible?
Almost without realizing it, we have gone on to unlock the mobile phone by using the fingerprint, to open a bank account by means of a facial picture or to identify ourselves through the voice and with it the question has become: how do they do it?
How do biometric Identity verification technologies work?
It therefore seems necessary to have a greater knowledge of the basis and performance of the biometrics-based recognition technologies that will accompany us in the future in order to be aware of the opportunities they provide and also of the possible risks that may be associated with their non-legitimate use.
There is a basic principle applicable to any way of present or future verification: any physical or behavioural trait susceptible to be captured and mathematized is a strong candidate for use in a biometric process. There are many traits that are currently used: fingerprint, face, iris, voice, vascular pattern or DNA and even the analysis of behaviour in certain situations such as signing, walking or gestures
Once any of the biometric traits described above have been captured with an electronic device (a camera, a microphone, a fingerprint sensor…) it is possible to extract a sort of features that best represent each of them.
Once any of the biometric traits described above have been captured with an electronic device (a camera, a microphone, a fingerprint sensor…) it is possible to extract a sort of features that best represent each of them.
Until the recent irruption of deep learning, the process of selecting and extracting features was the fundamental pillar of the success of biometric systems based on machine learning, investing a large part of the time in the selection of features with two fundamental requirements: they must represent the biometric trait in a univocal way and also provide the greatest possible separation between different individuals.
Each application has its own requirements: if we need to design a system that must be able to recognize whether a voice note belongs to a man or a woman, the fundamental frequency will play an important role thanks to its discriminatory power (higher in women than in men), however if all the voice samples correspond to men or women this characteristic would remain in the background in favour of other more suitable ones.
When we have selected the features that best represent each biometric trait, it is time to give way to the machine learning processes.
Machine Learning Process in biometric identity verification
This concept, now so present in our daily lives, includes a number of algorithms whose purpose is to lead machines to make “intelligent” decisions about problems for which they had not been explicitly programmed: to decide whether a completely new voice memo belongs to a man or a woman after having been trained with hundreds of voice memos from different people.
Classically, the algorithms used in machine learning processes are grouped into three fundamental families:
- Supervised learning: In this case the system is trained with labelled examples, i.e. the desired output for each case is indicated so that it is possible to establish decision boundaries for each class
- Unsupervised learning: In the second case, it is the machine itself that will have to look for classification patterns to establish the boundaries.
- Reinforcement learning: The training process is based on trial-error mechanisms, so the machine will tend to carry out those actions that provide the maximum benefit (or the greatest reinforcements).
This was broadly the method used in biometric verification processes until about five years ago when everything changed…
The access to large databases available on the Internet and the cheapening and improvement of hardware for intensive computing (GPUs) brought back to the fore the neural network architectures that had previously given discrete results.
The access to large databases available on the Internet and the cheapening and improvement of hardware for intensive computing (GPUs) brought back to the fore the neural network architectures that had previously given discrete results.
With the paradigm shift, it is no longer necessary to be an expert in each specific biometric application or to spend long hours experimenting with the most appropriate feature sets for each case: deep neural networks are capable of creating layers of data representation with different levels of abstraction, from low-level features to more general ones.
Deep Learning and Neural Networks applied to biometric identity verification
The concept of deep learning involves some complexity but a concrete example makes a first approximation easier to understand.
Let us imagine that we face the problem of evaluating whether a facial image corresponds to a man or a woman and for this, we are going to use a deep neuronal network. The first thing we need is a database large enough to represent the different facial profiles that the system can face and start the training process.
Leaving aside the setup process of the network architecture and focusing exclusively on its performance, the different layers in which the system is structured will create representations of data with different levels of abstraction: those of low level will focus on features such as oriented edges or shadows, the following in others of greater complexity such as textures and basic forms and finally those of higher level in complex forms. The output of each layer will serve as an input to the next, facilitating and optimizing the learning process.
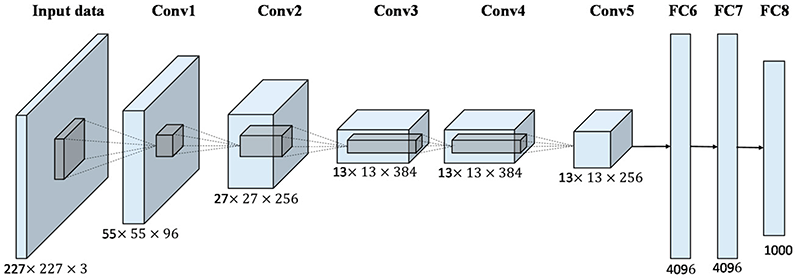
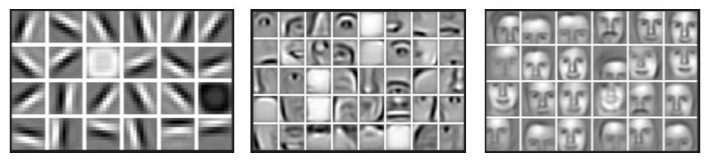
Once the deep neural network has reached the maximum possible learning point (in our case the greatest discriminatory power between genders) the training process will be finished and it will be possible to start using it to make predictions.
The facial image that we want to classify as masculine or feminine will cross the network extracting all its characteristics and finally it will be labelled as belonging to one or another group.
The methods based on deep learning have led to a remarkable increase in the performance of biometric systems, improving their reliability and security and this is why they are the current industry standard.
In Mobbeel we are fully aware of this circumstance and from the innovation department we carry out constant research into deep learning technologies to apply them to all our products. May the magic continue!

I am Head of Mobbeel’s Innovation Department and I work together with the rest of the team in the research and development of biometric and identity verification technologies for the continuous improvement of our products.
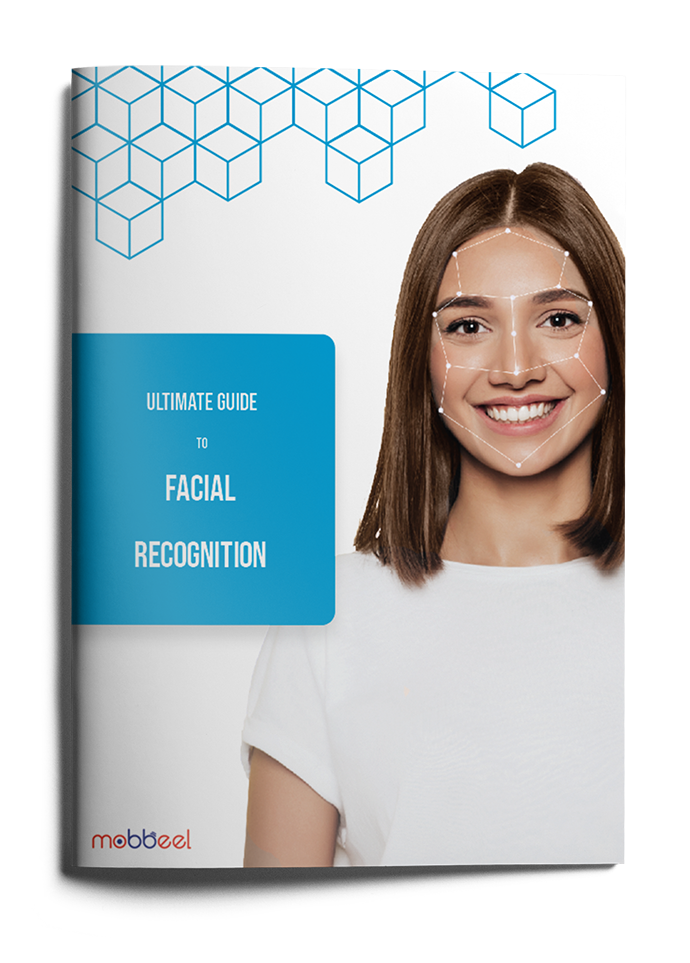
Discover what is face recognition
- Learn about the history of facial recognition. Origins and evolution.
- Learn how facial recognition systems work.
- See the different uses and applications.
- Understand the different implications of the technology in our society today.
- Discover the challenges in cybersecurity.
- Learn about the regulations involved in biometric processes.
Discover what is face recognition
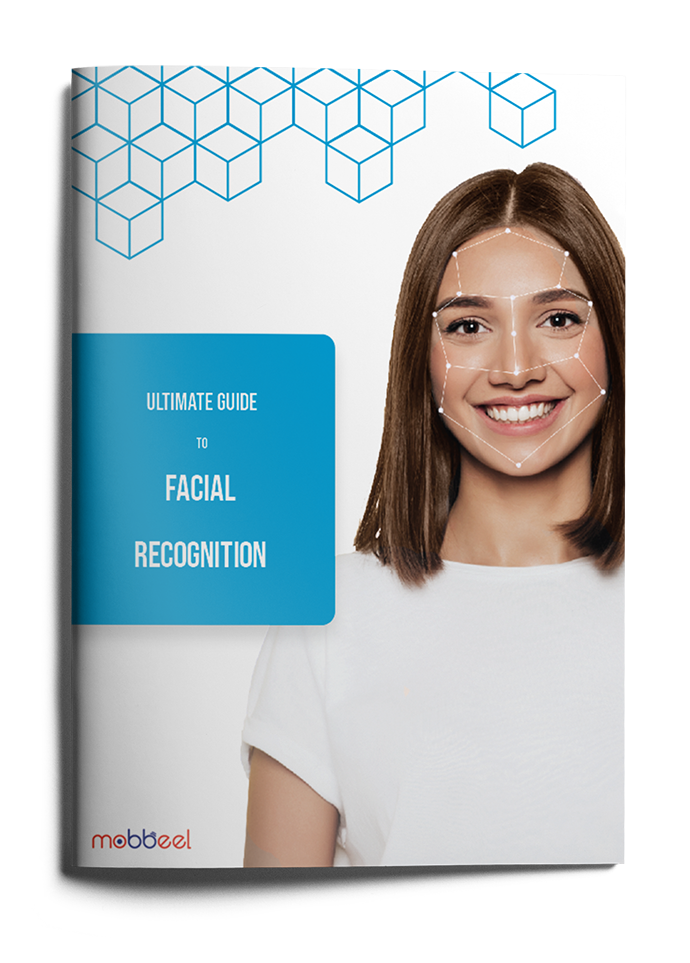