The proliferation of artificial intelligence and the ease of access to tools capable of altering the truth has given rise to one of the most shocking and worrying digital manipulation techniques: deepfakes. Deepfakes are an artificial intelligence technique that enables the creation of fake videos, images, and voices that show individuals saying or doing things they never said or did. Although until recently, the most widespread version was video, voice deepfake is gaining relevance day by day.
The first major milestone that brought the possibility of generating voice deepfakes to the general public was the launch of the Adobe Voco program in 2016. This program used artificial intelligence (AI) to imitate a person’s voice from a short recording. Nevertheless, the project was cancelled after realising that it could be used to create misleading and dangerous voice fakes.
Since then, advances in neural network technology have enabled increasingly realistic and convincing voice deepfakes. Nowadays, we can find several applications and online tools that use this technology to create voice fakes.
For instance, Microsoft launched an AI model called VALL-E. This model can replicate the human voice with only a three-second recording.
This reality has led us to analyse what a voice deepfake is, its impact, and how important it is to have detection models capable of identifying whether an audio clip has been synthesised.
What is a voice deepfake?
A voice deepfake is an artificial intelligence technique that aims to create a voice model capable of replicating an actual person’s voice (speaker).
This model should be trained with voice recordings of the speaker. Once trained, it is ready to generate a synthetic voice that sounds like the original person’s one. To do so, deep learning and natural language processing techniques are used to analyse the unique patterns and characteristics of the voice. Some of these characteristics include pitch, speed, cadence, and accent. Artificial intelligence uses the recordings to render the authentic-sounding voice, generating a new audio recording that mimics the speaker’s voice.
An example of this artificial voice simulation is the deepfake of Eminem’s voice that David Guetta played at one of his concerts. The DJ combined two generative artificial intelligence tools to create an Eminem-style verse and played it with Eminem’s deepfake voice.
But how is a voice deepfake created?
The creation of a voice deepfake is achieved by using AI-powered text-to-speech (TTS) technology. For a long time, two approaches have been used: concatenative TTS, which uses audio recordings to create libraries of words and sounds used afterwards to form sentences, and parametric TTS, which uses statistical speech models to recreate voices.
Technicalities aside, we can point out that today, with just a few minutes of recorded speech, audio datasets can be created and used to train an AI speech model that can read any text in the target voice.
Wavenet and Tacatron, to name a few, are some of the tools that can generate voice clones.
Elements for creating a voice deepfake
Tools such as Wavenet and Tecatron use three main elements:
- The original recorded voice. The recording must have sufficient quality to sample the unique characteristics of the speaker’s voice.
- The neural network. This network analyses the patterns and characteristics of the original voice and learns to imitate them. It is trained using deep learning algorithms and a large amount of audio data of male and female voices and can condition the network to the speaker’s identity.
- The generative model. It is in charge of generating the new audio recording.
Process of creating a voice deepfake
The process for the generation of this type of deepfake is as follows:
- The speaker audio recording is taken and divided into small audio samples.
- These audio samples are fed to the neural network, which analyses them and learns to imitate the characteristics of the original voice.
- Once the neural network has learned the patterns of the original voice, the generative model takes a small audio sample of the original voice and creates a new recording.
- The result is a fake voice recording that mimics the voice of the original person.
- In addition to generating speech, these tools can mimic sounds like breathing and mouth movements.
AVSspoof Challenge: the leading database against voice spoofing
Just as there are tools and programs to generate fake voices, initiatives aim to fight this increasingly widespread practice.
Challenge AVSspoof is one of them. Its main goal is to launch challenges for companies specialising in the field to analyse speech processing problems related to voice spoofing and design measures to combat it.
AVSspoof Challenge 2021
AVSspoof 2021 was the last challenge launched. It aimed to promote progress in reliable automatic speaker verification and deepfake detection in more realistic and practical scenarios.
For this purpose, telephone channels were to be simulated in which voice data was to be encoded, understood, and transmitted. At the same time, the acoustic propagation in physical spaces had to be analysed by creating sentences with the voice of a target person.
The challenge consisted of three tasks where each team had to detect a specific type of voice attack: logical access (LA), physical access (PA), and deepfake (DF).
- Logical access (LA): the objective was to study the robustness of the solutions against compression variations, packet loss, and other artefacts derived from bandwidth, transmission infrastructures, and variable bitrates issues.
- Physical access (PA): concerned with replay attack detection in different environments.
- Deepfake (DF): concerned with voice conversion (VC) detection and text-to-speech (TTS) synthesis over compressed audio. This task aimed to evaluate the robustness of spoofing detection solutions when used to detect manipulated speech data.
Challenge conclusions
Challenge results indicated that the robustness of spoofed audio detection is substantially improved when deepfake techniques are employed.
Furthermore, the study confirmed that non-verbal intervals could influence fake voice detection, especially in logical access and deepfake tasks.
Mobbeel used the challenge data to conduct technical tests. The results are competitive with those of the challenge participants and indicate we are at the forefront of deepfake detection technology, having an EER of 22 on the deepfake evaluation set.
How to detect voice deepfakes with biometrics
As the AVSspoofing challenge points out, detecting voice deepfakes is a constantly evolving problem. Nevertheless, some technologies can uncover these fakes, like voice biometrics.
Voice biometrics is an authentication method that uses a person’s unique voice pattern to verify their identity. This pattern includes characteristics like the tone of voice, intonation, speed of speech, and pronunciation.
To use voice biometrics, a person’s voice should be captured with text-dependent or text-independent voice recognition. After that, its unique characteristics are analysed along with the length of the words.
These characteristics are compared with a previously recorded voice profile stored in a database to determine the person’s identity.
Detection methods
Several methods can be used to detect audio deepfakes with biometrics:
- Spectral analysis. It consists of an audio signal analysis to detect voice patterns.
- Deep-learning algorithms that analyse an individual’s voice and recognise unique characteristics that are difficult to replicate in deepfakes.
- Detection of artefacts. They are digital traces found in voice deepfakes because they fail to simulate all the variables accurately of an actual voice recording. Thus, a deepfake may contain breaks in the voice or background noises different from those expected in authentic audio.
In addition to the above methods, this kind of biometrics allows you to compare voices with other voices and use those voices as a dataset to feed a deep-learning algorithm.
The type of biometrics we have mentioned so far would correspond to passive liveness testing, as they do not require any additional action or behavioural change from the user beyond using their voice. Additionally, complementing the passive liveness tests, an active liveness test could be performed that requires the person to do a system-requested action to be identified, such as pronouncing a phrase randomly generated from a list of predefined words.
How does Mobbeel R&D detect voice deepfakes?
Our R&D&I department uses an architecture that combines several machine learning models. These models include convolutional and deep learning networks and have been trained to detect attacks of various types, including deepfakes.
By combining several models, the decision as to whether a voice is a forgery does not rest with a single model, adding extra reliability to the detection.
Detection of artificial voices of the VALL-E model
The VALL-E model is Microsoft’s new language model for text-to-speech synthesis (TTS). This tool only needs a three-second recording to imitate a human voice.
Nevertheless, although the model can be considered an improved version of deepfake, the voices still sound robotic, so according to our R&D department, they are easily detectable.
Do not hesitate to contact us if you want to know what more our R&D&i department can achieve.
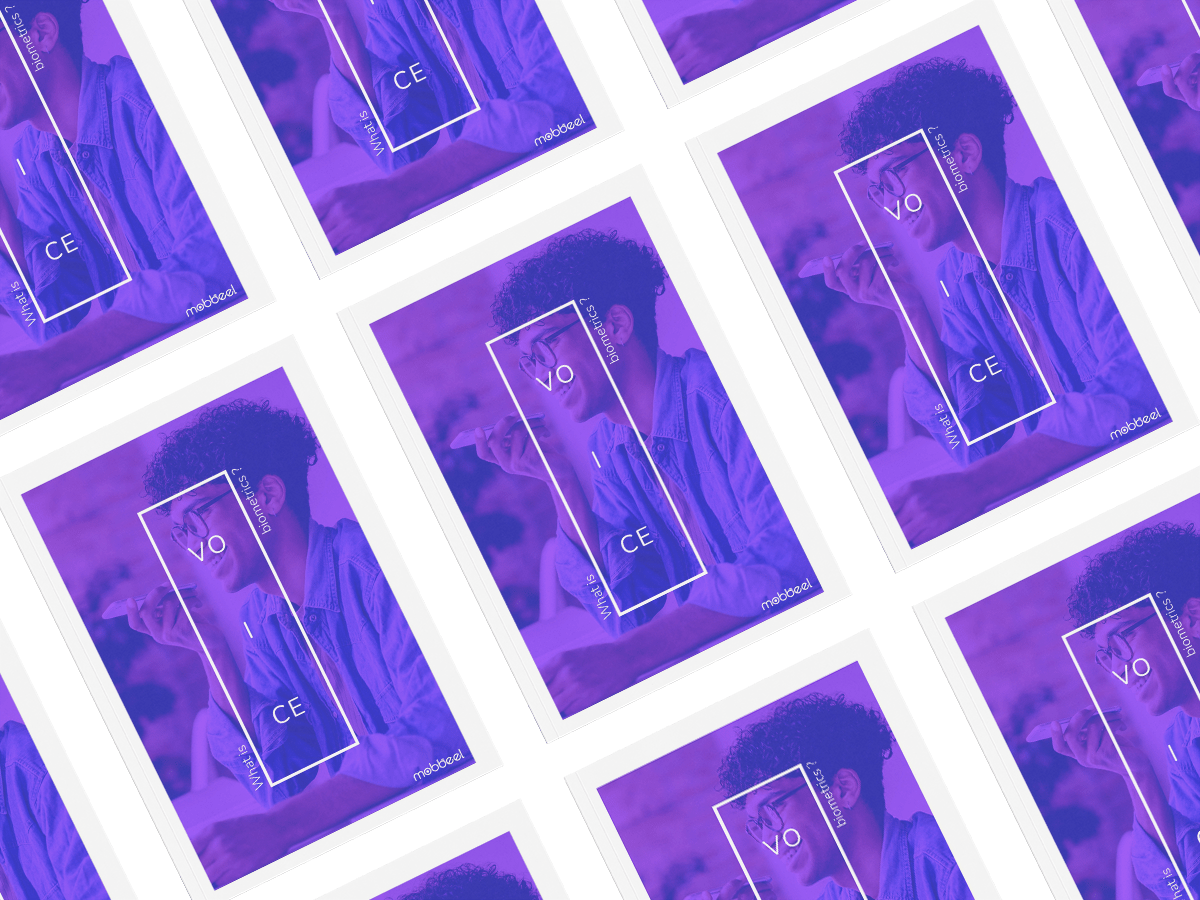
GUIDE
Know your customers through their voice
Thanks to advances in AI and language processing, voice biometrics has become a valuable tool for identification in multiple use cases. The uniqueness and non-replicability of each person’s voice make it an exceptionally secure and reliable method of authentication.
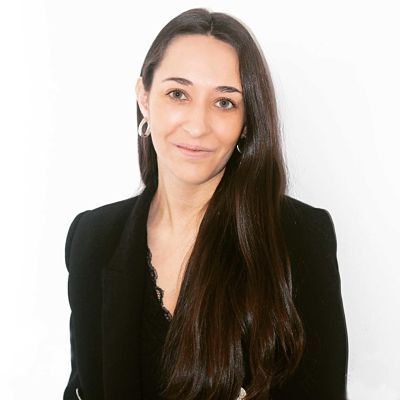
I am a curious mind with knowledge of laws, marketing, and business. A words alchemist, deeply in love with neuromarketing and copywriting, who helps Mobbeel to keep growing.